Making sure you're not a bot!
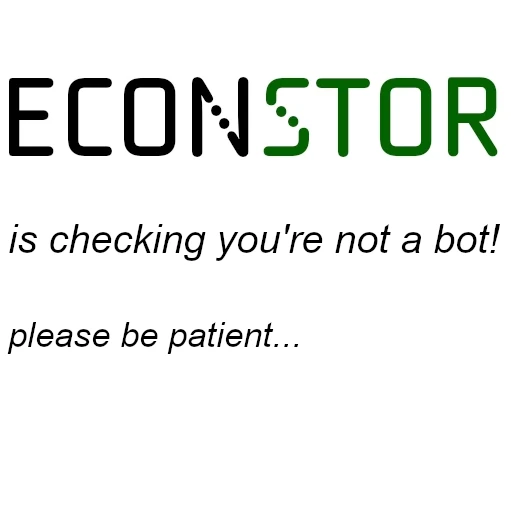
Loading...
Why am I seeing this?
You are seeing this because EconStor has set up Anubis to protect the server against the scourge of AI companies aggressively scraping websites. This can and does cause downtime for the websites, which makes their resources inaccessible for everyone.
Anubis is a compromise. Anubis uses a Proof-of-Work scheme in the vein of Hashcash, a proposed proof-of-work scheme for reducing email spam. The idea is that at individual scales the additional load is ignorable, but at mass scraper levels it adds up and makes scraping much more expensive.
Ultimately, this is a hack whose real purpose is to give a "good enough" placeholder solution so that more time can be spent on fingerprinting and identifying headless browsers (EG: via how they do font rendering) so that the challenge proof of work page doesn't need to be presented to users that are much more likely to be legitimate.
Please note that Anubis requires the use of modern JavaScript features that plugins like JShelter will disable. Please disable JShelter or other such plugins for this domain.
This website is running Anubis version v1.20.0-21-g510835d
.